Blog Insights
Experts Weigh In: Applying Artificial Intelligence (AI) and Machine Learning (ML) for Government
I find the rapid developments in Artificial Intelligence (AI) and Machine Learning (ML) thrilling, with endless applications for our Forum One partners, but I know from discussions with my counterparts in government agencies that AI and ML can also be mystifying, and even scary.
We love to extend learning at Forum One, so I was excited to be able to bring two experts at the forefront of new digital applications for government together for an open conversation, How & When to Create Custom AI/ML Solutions in Government.
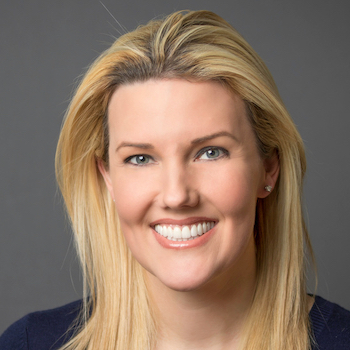
Alexis Bonnell is the former Chief Innovation Officer of USAID, where she had the privilege of working with thousands of innovators, inside and outside of USAID who are changing the world and helping millions of people become self-reliant. In 2018, USAID received a 10/10 in Innovation in the “Results for America” study of Federal Agencies. She previously served as the Chief of Applied Innovation & Acceleration in USAID’s U.S. Global Development Lab, one of the premier Innovation Labs in the development industry, that she helped create in 2014. She serves as a key spokesperson and “Innovation Evangelist” across the industry driving innovation and modernization.
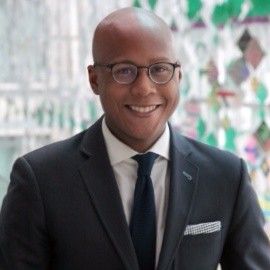
Jay Finch is the founder and CEO of Polydelta, a platform that helps to deliver public sector outcomes with AI. Jay has advised U.S. Federal agencies on digital transformation strategy as the 18F Director of Agency Partnerships and Senior Advisor at the Technology Transformation service within GSA. He was recognized in Forbes magazine’s “30 under 30” for law and policy and as a Rising Star by Federal Computer Week. Jay earned a B.A. from Villanova University, an MPA from the Harvard Kennedy School, and an MS from the Stanford Graduate School of Business as a Sloan Fellow.
Catch highlights of our conversation paraphrased below, or listen back to our entire conversation anytime. And if you have questions or ideas about getting started or expanding AI and ML in your practices, reach out! We’d love to explore with you: inquiries@forumone.org.
What’s a recent project that illustrates the value of AI or ML to you?
Alexis: The state of Hawaii mapped its infrastructure, with overlays of potential climate impacts and considerations of vulnerable populations, allowing them to run scenarios and test hypotheses for different preparedness measures. If there’s historic rain, who feels that impact the most? I appreciate that this is government being curious and forward-looking.
Jay: A tool that used machine learning to help college students discover internships in the conservation field. This is information that is available but not necessarily accessible to the student population without a significant amount of time, connections, and research. The tool was developed to have a conversation with a student, show them a few internships, and then learn from the student’s feedback what types of opportunities and locations would best fit them. It was then able to present a longer, curated list of opportunities for the student to explore.
What types of projects are well-suited for AI?
Alexis: We look at where there are pain points—internally, what is creating “toil” on employees, who could otherwise be freed up for more human challenges? Externally, what is exacerbating “time poverty” among users? Things like form processing, form filling, and administrative steps. And, look to projects that fill a void, or provide a service that can’t otherwise be offered. For example, the government of San Jose created an after-hours chatbot, available in multiple languages, to serve what they recognized as a need from Spanish- and Vietnamese-speaking populations who could not take time off work to meet at a government office during business hours. The chatbot offered service at a time when none was offered previously. And, by offering an opportunity to learn from the questions and needs expressed through the chatbot, the government could learn more about how to tailor their services for populations they may not have heard from otherwise.
Jay: Some basic applications where AI can deliver include recommendation systems—should I respond to this email or not, what product should I purchase next?–these are well-understood applications of AI with meaningful results. Also prediction services, for things like weather, or event attendance, can help users predict that something seen in the past will happen again. Last, AI is helpful for handling non-uniform inputs. Think of Google search, and natural language processing; the ability to make sense of varied phrasing or word choice, that in aggregate can be processed.
What are the pitfalls of AI, or scenarios for which it isn’t well-suited?
Alexis: AI is not a set-it-and-forget-it tool. You really have to want to be on a learning journey and be willing to see what it shows you about your programs and processes. The process is always: gain insight, pivot; gain insight, pivot.
Also, people worry about AI introducing bias, so they avoid using it. But in a lot of scenarios applying AI to existing data can actually reveal bias that was already there.
Jay: There are certainly times when AI can deliver worse results than more traditional methods. The rush to machine learning can overlook the benefits of regular rule-based coding. If you can simply estimate, or apply a policy, such as income at X level being taxed at Y rate, you don’t need to apply machine learning.
Also, data can become stale, or skewed, and there are risks to relying on a model for too long without adjustments. In some ways you have to treat machine learning like an employee—training, and supervision are required to accomplish more significant tasks or tasks that change over time.
How can agencies get started?
Alexis: First, don’t be afraid to start at a personal level, to just explore AI. Two tools you can use are Google’s Teachable Machine and Chat GPT. Be curious and have fun with it to start! Then, be really intentional about educating yourself and others. At work, ensure all players are on the same page. At USAID we had procurement teams, and legal folks in the room to pressure test, and make sure we were talking about the same things.
Jay: We have a clear process that starts at a human level. Think of humans first, and then, what’s your north star—what do you want to accomplish with this project? What’s your theory of change? Continue to revisit those ideas at every step—are we aligned with our north star? Does the project support our theory of change? Then, ideate and socialize broad ideas. Start small and scale up, never being afraid to go back to the first steps and check your assumptions.
Again: if you have questions or ideas about getting started or expanding AI and ML in your practices, reach out! We’d love to explore with you: inquiries@forumone.com.